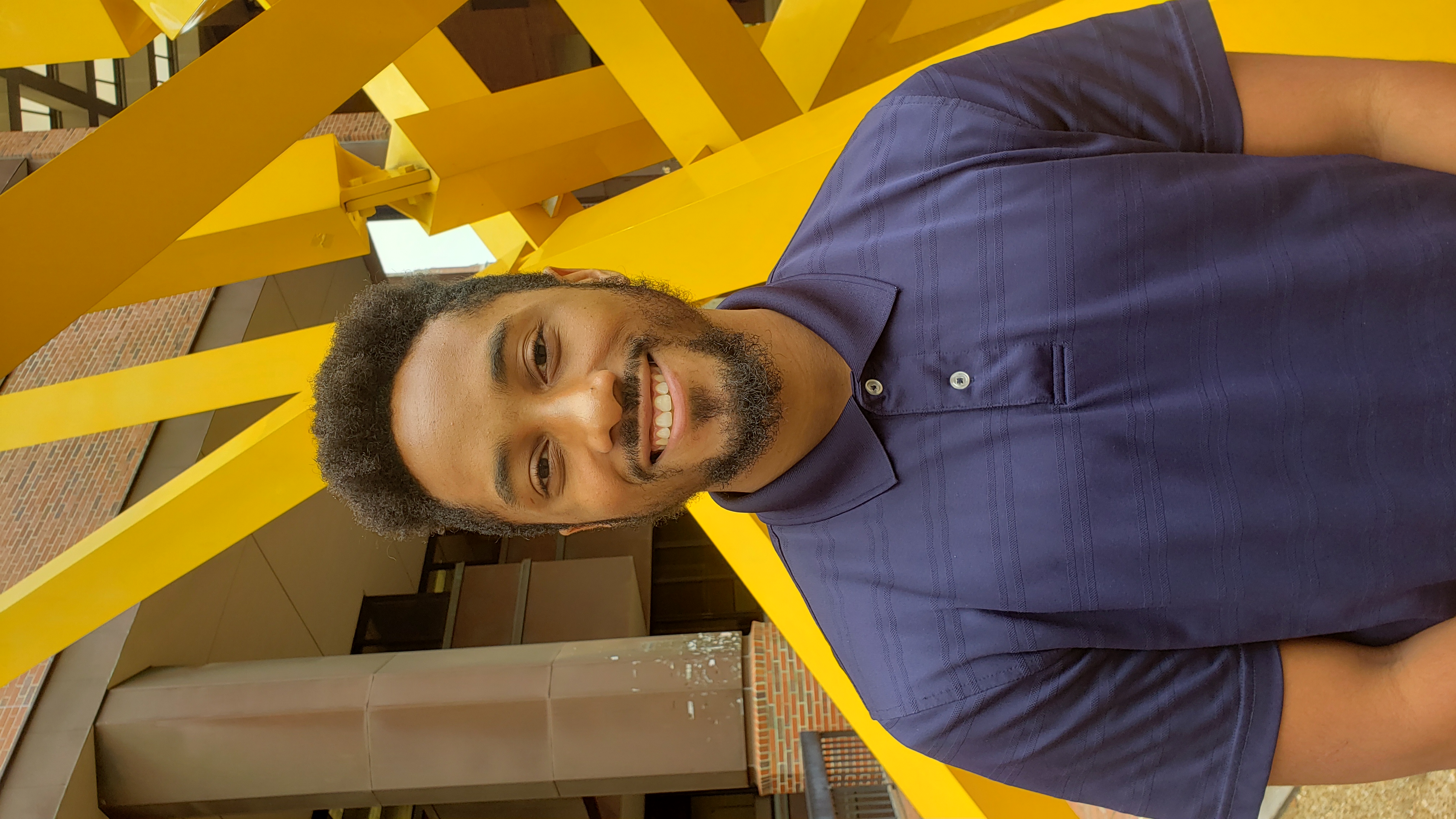
The goal of this study was to assess the validity of using head-mounted displays in a learning environment compared to conventional technologies (in this case a laptop).
I graduated in 2018 with a Bachelor's of Science in Computer Science from the Unversity of Maryland, Baltimore County. I am a current graduate student at the University of Florida, studying Human Centered Computing. My research interests include studying how to better understand and make more efficient human-computer interactions within the scope of automated systems and processes.
PhD Research in Human-Centered Computing
Sustained Research in Artificial Intelligence
Internship
Work experience
The goal of this study was to assess the validity of using head-mounted displays in a learning environment compared to conventional technologies (in this case a laptop).
This preliminary study explored the effects of one's native language on their ability to pick up programming languages. We studied two participant categories. English as a first language and Spanish as a first language but still able to speak English. This motivation here is that most programming languages are syntically based in English but this could affect one's ability to understand and pick up the language when learning programming for the first time. All participants never programmed before and used Scratch, a block-based programming language to complete a set of tasks. We found that participants who spoke Spanish as a first language took more time to take action when moving the blocks as compared to first language English speakers, which we believed could be a representation of a lack of understanding. However, those Spanish-speaking participants also seemed to explore the blocks more. The work here will continue with participants that do not speak English at all to assess their capacity to understand these English-based tools.
TBA
TBA
K. McNamara, I. Sherman, F. Tavassoli, J. D. Louis and J. E. Gilbert, "On media and disinformation: Examining viewer judgment of political video authenticity," 2021 IEEE International Symposium on Technology and Society (ISTAS), 2021, pp. 1-9, doi: 10.1109/ISTAS52410.2021.9629120.
P. G. Feijóo-García, K. McNamara and J. Stuart, "The Effects of Native Language on Block-Based Programming Introduction: A Work in Progress with Hispanic Population," 2020 Research on Equity and Sustained Participation in Engineering, Computing, and Technology (RESPECT), 2020, pp. 1-2, doi: 10.1109/RESPECT49803.2020.9272513.
Sherman, I., Bowers, J., McNamara, K., Gilbert, J.E., Ruiz, J., & Traynor, P., (2020) Are You Going to Answer That? Measuring User Responses to Anti-Robocall Application Indicators, In Proceedings of the ISOC Network & Distributed Systems Security Symposium (NDSS).
Pedro G. Feijoo-Garcia, Keith McNamara, Jr., and Jacob Stuart. 2019. Work inProgress: Native Language Consistency Effect on Learners’ Introduction to BlockBased Instructional Technologies. In Proceedings of the 20th Annual SIG Conference on Information Technology Education (SIGITE ’19). Association for Computing Machinery, New York, NY, USA, 147. DOI: https://doi.org/10.1145/3349266.3351368
In many situations in life, humans must take the knowledge they gain in one environment in use it in another. One goal for artificially intelligent agents is to get them to do the same when learning the environments they are in. As a member of the MAPLE Lab at UMBC, I worked on generalizability with artificially intelligent agents. More specifically, my research involved knowledge transfer across different domains.
The classic GridWorld domain, a navigation-based domain that works as a grid. The agent can move in the north, south, east, and west directions. Along with another student, I helped to design an object-oriented approach to this domain in which every piece of the domain was an object. This would serve as a good training domain for incoming students to learn the basics domain design and agent learning.
Often, much is not known about a domain when in it, so the agent must learn as it exists. For example, someone in a dark room must find the lights and figure out what they are touching as they navigate. One of my projects involved trying to develop a partially observable domain with the same concept. This domain was called RockSample, based on a previously developed domain. It involved an agent that traversed a rock domain and must collect rocks with certain value. The qualities of the rocks were not known until the agent made contact with them.
Abstract Markov Decision Processes, or AMDPs, are Markov Decision Processes with layers of knowledge abstracted out. More simply, the agent does not consider certain information until it completes some prerequisite task. As an independent study project, I compared the learning rates of different A.I. learning methods, such as QLearning, MAXQ, and AMDP methods. I also tested increases in scale of the domain to determine how the learning rate was affected by the increases in scale.
I worked in the Technology Services sector, in positions ranging from software documentaton, cybersecurity, and software engineering. The weekly software reports entailed my reviews of employee documentation regarding their software updates and progress. When I worked with software engineers, I was able to hand first-hand experience seeing the code development and documenting what processes were being developed. My most notable task during this internship was my work with the Microsoft Sharepoint feature within the company. I was able to provide insight and work/communicate closely with the developers working on the system. I would attend the group meetings with the developers and be a part of the conference calls which would facilitate the progress and updates of the project.
At the University of Maryland, Baltimore County, I worked within the IT infrastructure space as well as being a part of setting up computer labs and administration computers. My position mostly entailed image installation and troubleshooting. As a result, I needed to be fluent with the Windows and Apple operating systems and computer booting techniques.
Semester Project in Virtual Reality on the effects of climate change
A study focusing on leveraging attention in AR
Evaluating user response to various spam call warning designs
Send me an email.